What is Data Cleaning?
Data cleansing is an imperative part of data science. Functioning with corrupt data can cause many issues and difficulties.
Therefore it is vital to understand why data cleaning is essential for data enhancement, what factors affect your data quality, and how you can clean your data precisely.
Why Data Cleaning is Necessary?
Data cleaning might appear casual and boring, but it’s indeed one of the most crucial pieces of work that you need to do as a data science professional. Working with incorrect or poor quality data can be harmful to your work. Corrupt data can cause any brilliant algorithm to fail.
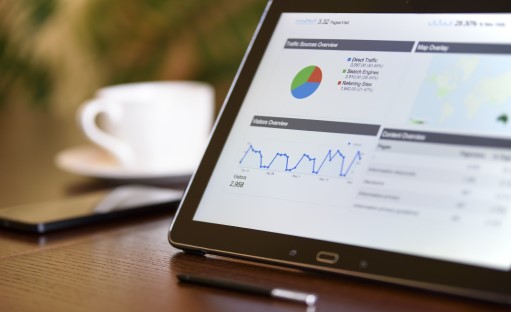
Whereas, high-quality data can provide excellent results even with a simple algorithm. There are many data cleaning techniques, and you must get familiar with them if you want high-performance for your system.
Keep a note that not all of your data is useful. Whether you are working on technology, education, healthcare, manufacturing, or any other domain, you must get rid of the unnecessary data that is sure to pile up in your system which lowers your data quality.
How to Determine Data Quality?
How valid is your data? The validity of your data is the degree up to which it obeys your requirements. Validity errors take place when the input method isn’t correctly examined. You might be using spreadsheets for data collection. You also might end up entering the wrong information in the cells of the spreadsheet. Therefore valid as well as verified data is a must for having a top-notch data quality.
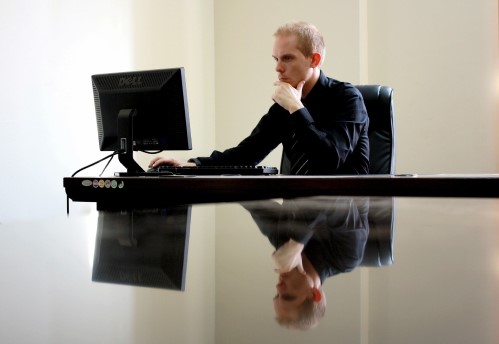
Steps to Clean your Data
1. Get Rid of Irrelevant Values
The foremost thing you must start with is removing useless pieces of data from your system. Useless data is the one you don’t need and is irrelevant to you. It might not fit the context of your subject.
2. Remove Duplicate Values
Values that are duplicates are similar to useless values. You don’t need them any which way. They will only increase the amount of data you have and waste your time and energy. These can be fixed with simple searches.
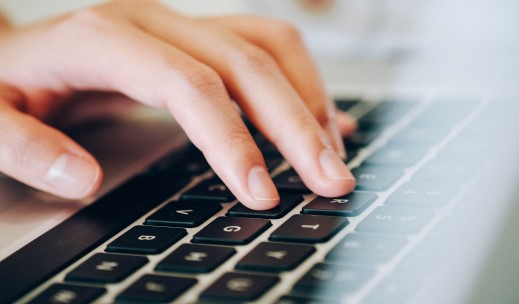
3. Avoid Typos (spelling mistakes)
Spelling mistakes, grammatical errors, or typos are a result of human error and can be present anywhere. Typos can be fixed through multiple algorithms and techniques. You can easily map the values and convert them into the correct spelling.
4. Check Data Types
All data types should be uniform across your dataset. A string can’t be numeric or a numeric can’t be a boolean.
5. Fix Missing Values
There always remains a piece of missing data. You just can’t avoid it. You must learn how to fix them to keep your data clean and free from errors. Ignoring loopholes such as missing values can turn out to be a costly mistake as it will reduce your data quality, and you won’t get accurate results. You can easily fix missing values by imputing relevant data.
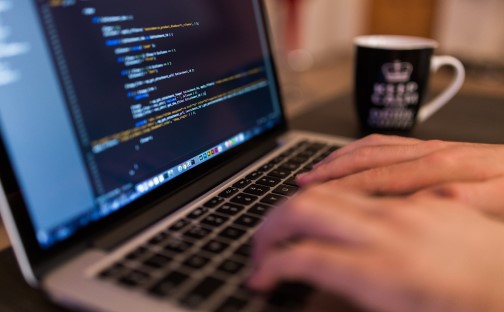